In Data We Trust
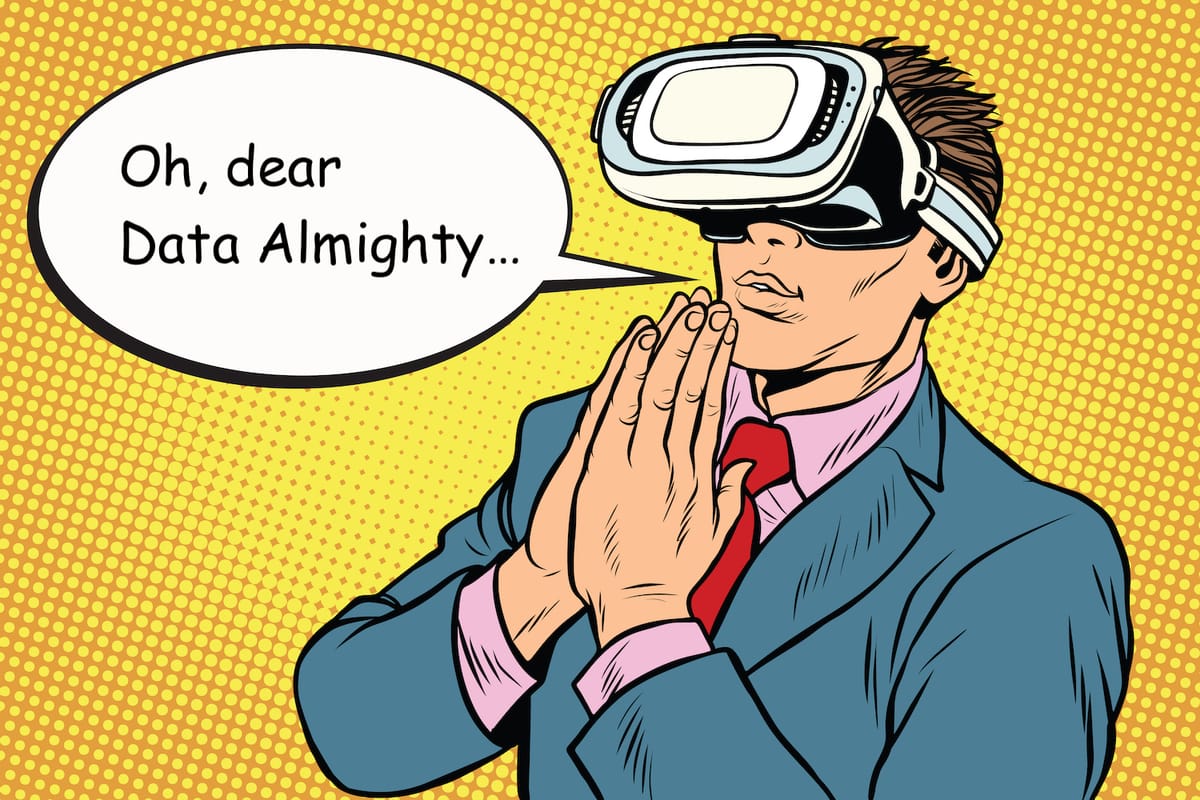
We have a natural tendency to believe what we see on our screen is the truth.
When engineers in Natanz nuclear site were sitting in their highly secure operations control room, they thought they were performing their day-to-day duties, carefully monitoring systems for any malfunctions.
Little did they know that their systems had been infected with Stuxnet - a computer worm, a cyberweapon - causing the fast-spinning nuclear centrifuges to tear themselves apart.
They blindly trusted their computer screens - but this malicious computer worm had changed the screens to make it seem like everything was normal. Causing a major hit to Iran's nuclear fuel enrichment program.
"What do hackers, fraudsters, and organized criminals have in common with Facebook, Google, and the NSA? Each is perfectly capable of mediating and controlling the information you see on your computer screens." — Marc Goodman, Future Crimes
It doesn't need to be always that exciting with such a sinister motive.
In marketing, we work daily with tools that seemingly provide precise data. In Google Analytics, we see exactly 10,398 visits to an article. In an A/B testing tool, we see that a new version is better by 3.24%. A keyword has an average monthly search volume of 1,400.
We like simple answers.
So marketing tools provide them to us.
What we see in a dashboard is considered reality.
But website analytics data is wildly inaccurate.
Due to cookie consent policies, ad blockers, and tracking prevention features in modern browsers.
Due to bots, tags not firing soon enough.
Due to people using multiple devices.
Due to internal traffic.
Reality? Pfff.
Then we have the celebrated A/B testing. A go-to activity for any data-driven project.
I used to be very uncritical of A/B and multivariate testing, but then I saw Craig Sullivan's presentation on "20 Simple Ways to Fuck Up Your AB Tests". Craig Sullivan is a well-known CRO expert. That presentation truly grounded me.
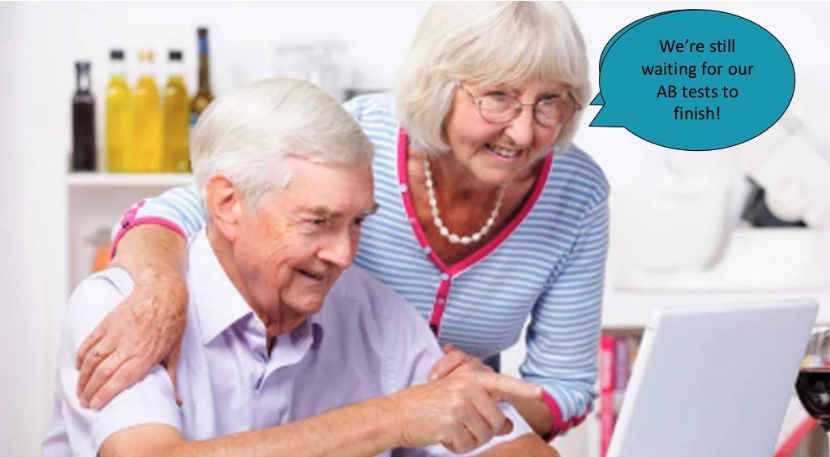
If people considered at least some of the points from his (now prehistoric) presentation, instead of blindly trusting what Google Optimize spits out, their data-driven efforts would have a much better impact.
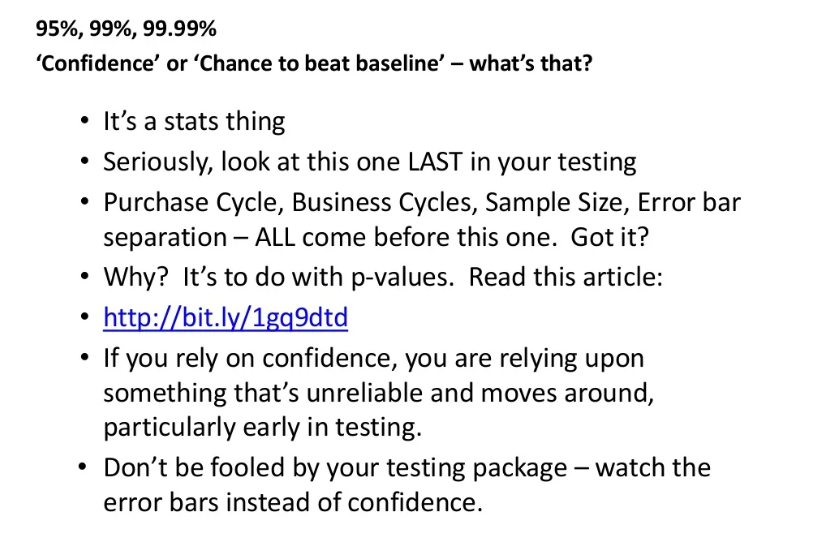
When you start digging deeper and getting a better understanding of proper testing, a period of disillusion kicks in.
When David Kadavy increased his conversions by 300% by doing absolutely nothing, he learned that A/B testing correctly takes tremendous energy.
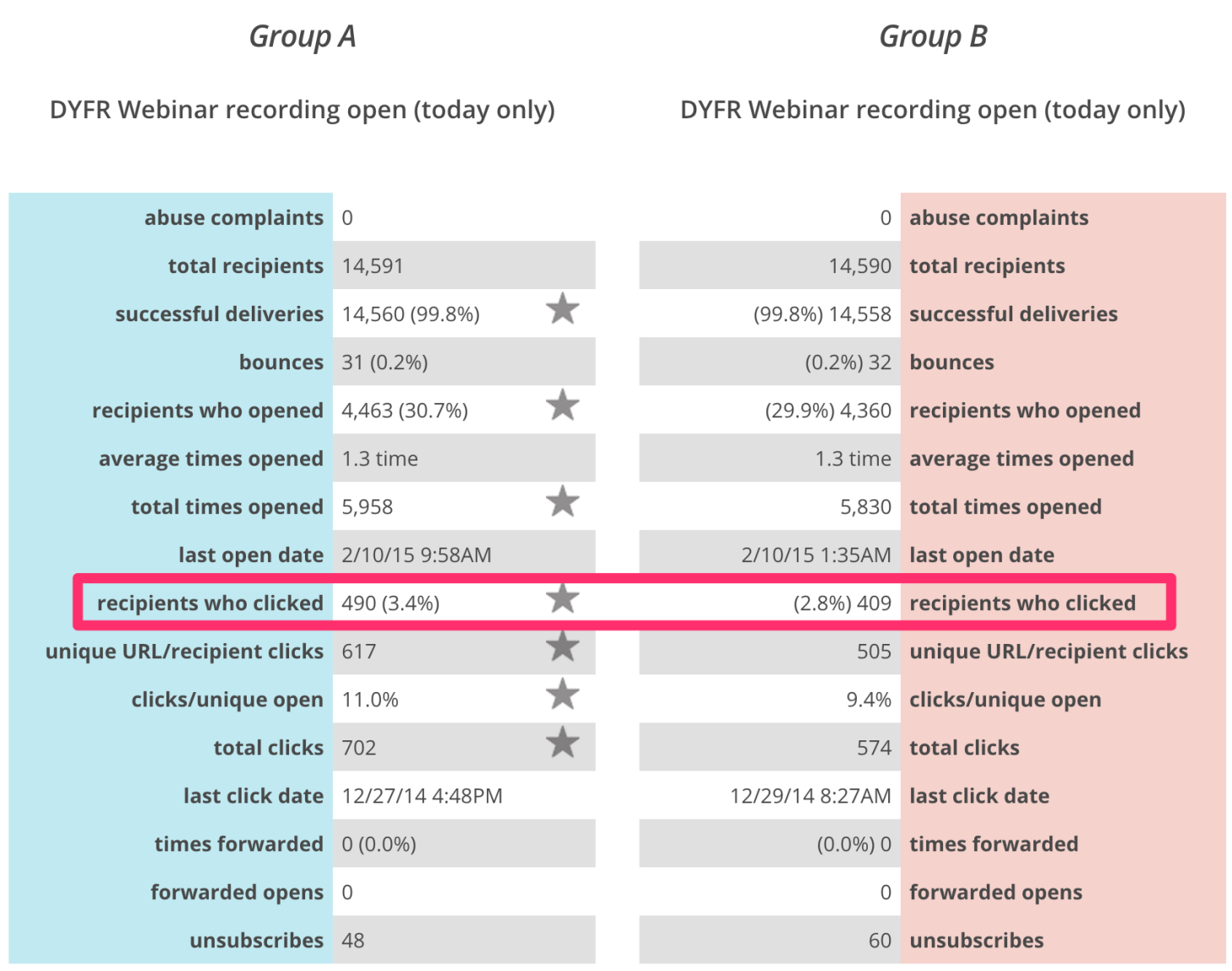
There are so many ways to make A/B testing a complete waste of time.
In SEO, it's not much better. Search volumes of keywords are often outdated and inaccurate. They are usually simple monthly averages that are not counting for seasonality.
On top of that, 15% of all Google searches have never been searched before and we have no data on them.
As SEOs, we like to turn to Google Search Console because it provides more valuable information for us than Google Analytics. Yet, GSC hides so-called anonymized queries - in the recent Ahrefs study, these instances of hidden terms accounted for 46.08% of all clicks!
That's a lot of data you don't see.
And it will likely only get worse and worse.
The list of things we cannot track at all grows as well.
There's the Google Discover feed that you can't track in Google Analytics.
There's Siri Suggested Websites on iPhones and in Safari browsers which you can't track or evaluate at all.
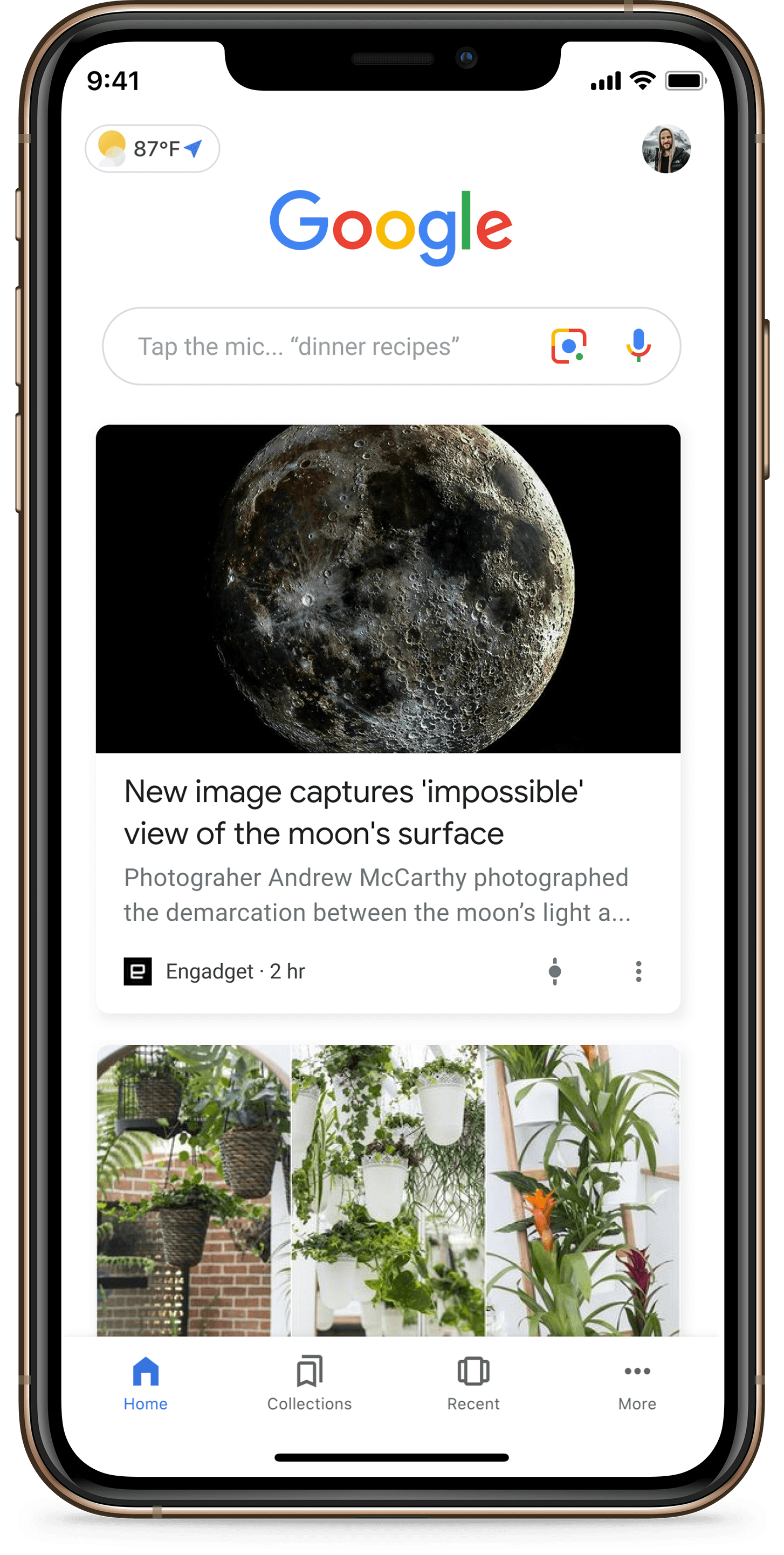
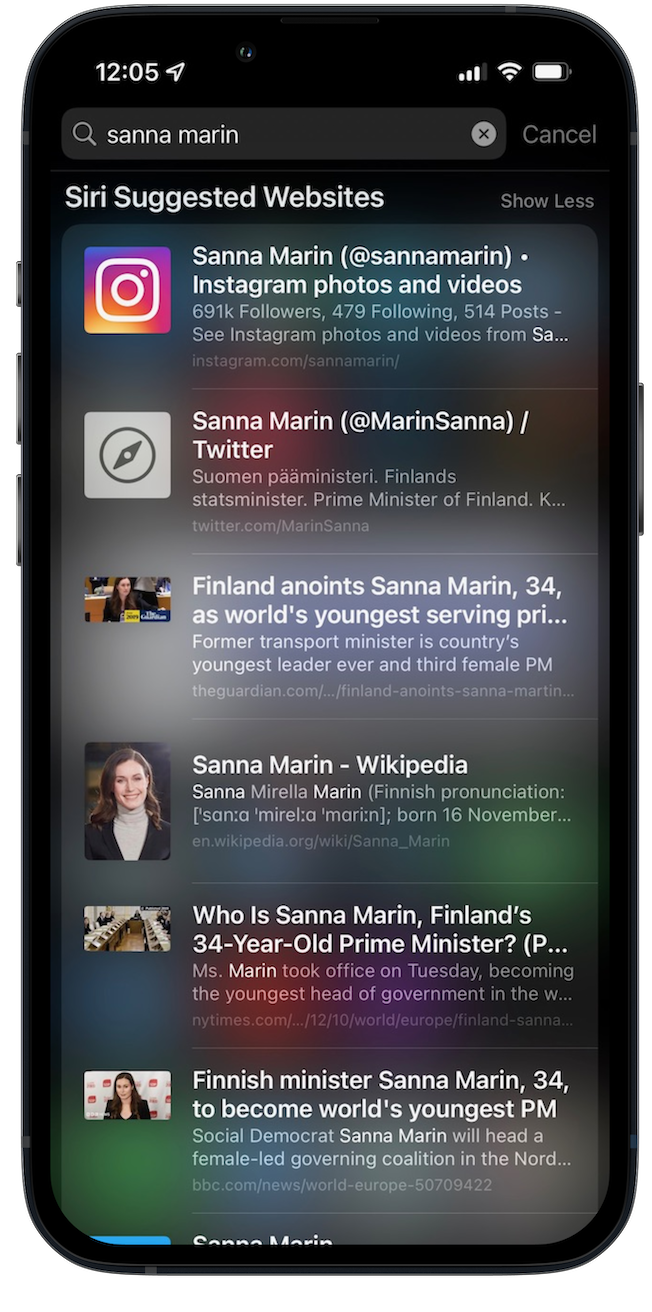
Google Discover and Siri Suggested Websites
And there's always been dark social - social shares that do not contain any digital referral information about the source.
We'll have less data and need to figure out how to work with what we have.
Maybe, we could extrapolate and try to calculate the unknown. In a similar way to Google Ads and its consent mode modeling - trying to pluck the number of conversions out of the air... oh, I mean, use proprietary and completely black-boxed modeling to calculate unconsented conversions.
Or maybe, we'll just use common sense more often and go back to the basics of marketing.
Do I think we should stop testing and stop looking at data? Not at all.
I think we really need to:
- Question data, be critical and want to know how metrics were calculated. Try to understand the level of error or uncertainty in a reported measurement.
- Just do it. Don't rely on data every time. Ask yourself frequently: It is better to invest tremendous effort into a correct A/B test, or run poorly set up A/B test with questionable results, or simply do it. You'll probably find out that in many cases, it's best to implement a change and see results, rather than waste time and resources with testing. At Ghost, when they wanted to improve their onboarding process, they decided to "JFDI" instead of testing - resulting in significant improvements with very little work:
This improvement gets us an extra 0.84 customers per day, and our average Ghost(Pro) customer Lifetime Value is currently about $200. Basic napkin maths – 365 days * ($200 * 0.84) – says that this change will be worth $61,000 in additional customers each year.
Not bad, for 30 minutes work. (source)
And more importantly, they can move on to improve their product further instead of waiting for the test to finish. The smaller you are, the more frequently you need to JFDI.
- Roll with the punches, but stay vigilant. Understand that you see only a small portion of the full picture in your dashboards, and don't be too obsessed with it. Know the limitations and try to use what you have in the best way possible. But don't be like the engineers at Natanz nuclear site blindly staring at the screen, thinking that's the only truth while the whole building is tearing up under your ass.